President Award Click here CAREER Click here Online Programmes Click here
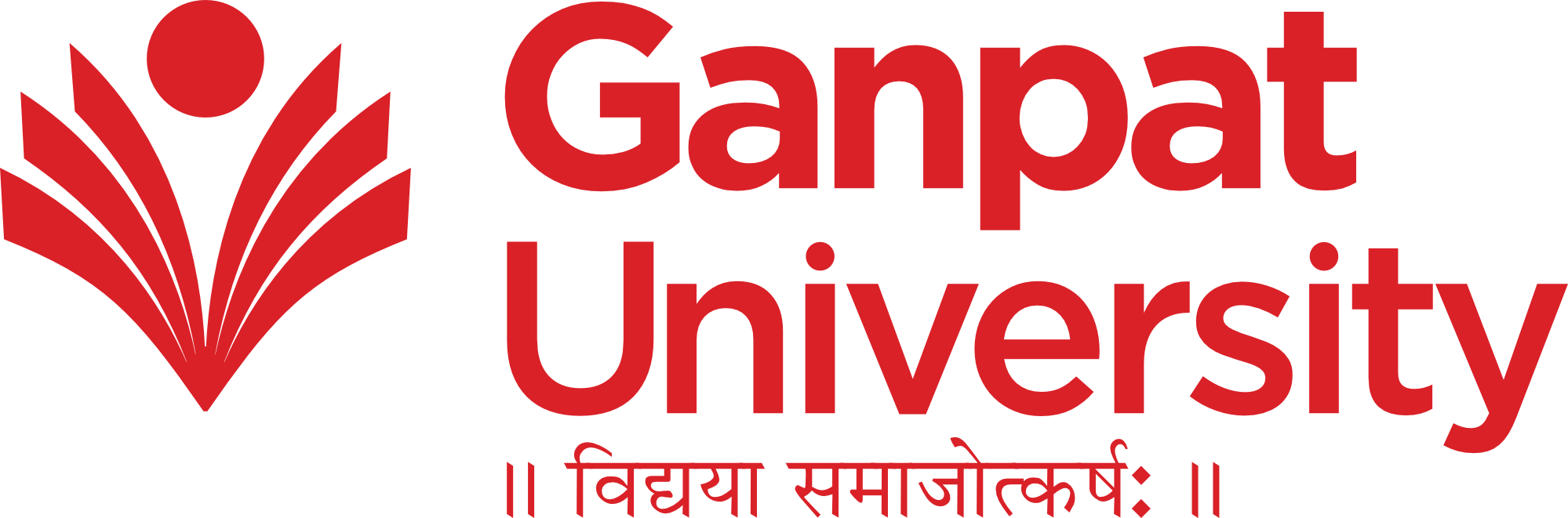
President Award Click here CAREER Click here Online Programmes Click here
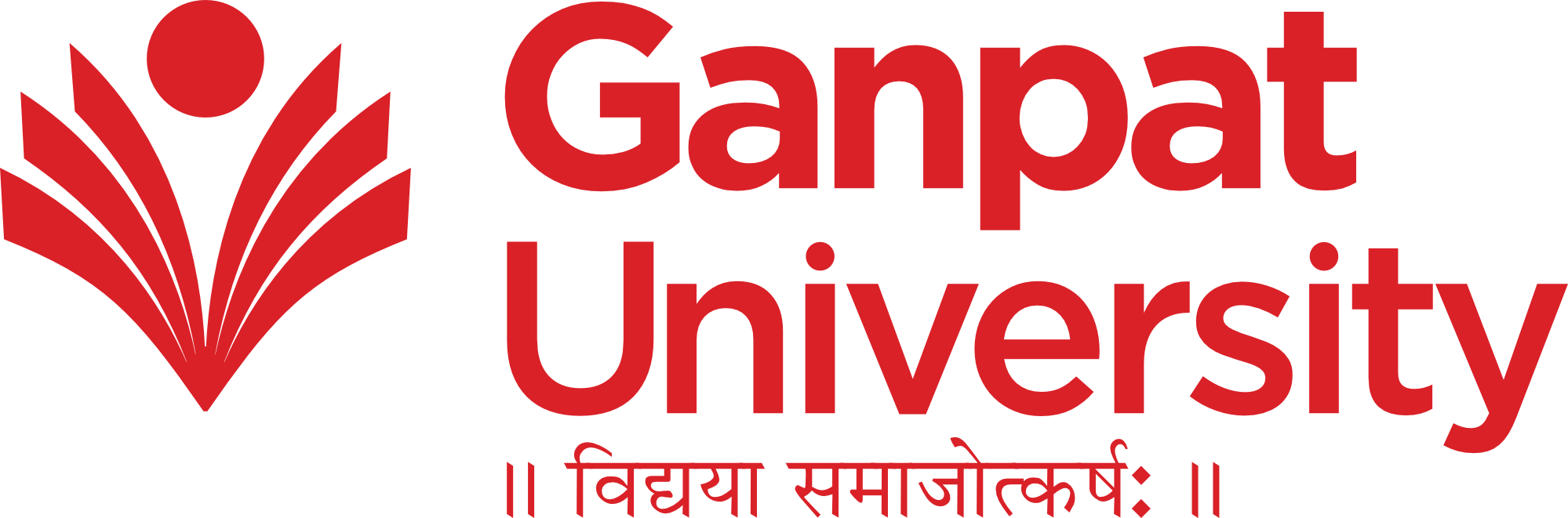
ADMISSION ENQUIRY - 2025
Data Mining and Warehousing
GANPAT UNIVERSITY |
|||||||||||||||
FACULTY OF ENGINEERING & TECHNOLOGY |
|||||||||||||||
Programme |
Bachelor of Technology |
Branch/Spec. |
Computer Science & Engineering (CBA/CS/BDA) |
||||||||||||
Semester |
VI |
Version |
1.0.0.1 |
||||||||||||
Effective from Academic Year |
2022-23 |
Effective for the batch Admitted in |
June 2020 |
||||||||||||
Subject code |
2CSE60E27 |
Subject Name |
Data Mining & Warehousing |
||||||||||||
Teaching scheme |
Examination scheme (Marks) |
||||||||||||||
(Per week) |
Lecture (DT) |
Practical (Lab.) |
Total |
CE |
SEE |
Total |
|||||||||
L |
TU |
P |
TW |
||||||||||||
Credit |
2 |
0 |
1 |
0 |
3 |
Theory |
40 |
60 |
100 |
||||||
Hours |
2 |
0 |
2 |
0 |
4 |
Practical |
60 |
40 |
100 |
||||||
Pre-requisites: |
|||||||||||||||
Basics of Probability & Statistics, Python/JAVA Programming Language, Database Management System |
|||||||||||||||
Learning Outcomes: |
|||||||||||||||
After successful completion of the course, the student will be able to:
|
|||||||||||||||
Theory syllabus |
|||||||||||||||
Unit |
Content |
Hrs |
|||||||||||||
1 |
Data Warehousing Basic Concepts, Data Cube and OLAP, Design and Usage, ETL, Implementation |
5 |
|||||||||||||
2 |
Data Mining Basics Importance, Functionalities, Classification, Architecture, Major Issues, Data mining metrics, Applications, Social impacts of data, Data Mining from a Database Perspective |
3 |
|||||||||||||
3 |
Data Pre-processing Descriptive Data Summarization, Data Cleaning, Data Integration and Transformation, Data Reduction, Data Discretization |
3 |
|||||||||||||
4 |
Association Rules Algorithms, Advanced Association Rule Techniques, Measuring the Quality of Rules |
5 |
|||||||||||||
5 |
Classification Basic issues regarding classification and prediction, Decision Tree, Bayesian classification, K Nearest Neighbour Algorithm, Associative classification, Statistical-Based Algorithms, Rule-Based Classification |
7 |
|||||||||||||
6 |
Clustering Similarity and Distance Measures, Hierarchical Algorithms, Partitioned Algorithms, Clustering Large Databases, Clustering with Categorical Attributes |
7 |
|||||||||||||
Self-Study Topics |
|||||||||||||||
Data Mining Applications, Mining Event Sequences, Visual DM, The WEKA data mining Workbench |
|||||||||||||||
Practical Content |
|||||||||||||||
Practical contents will be based on following concepts like Web Scraping, Text Mining, Implementation of Classification, Association Rule based and Clustering Algorithms |
|||||||||||||||
Mooc Course |
|||||||||||||||
Course Name: Data Mining |
|||||||||||||||
Text Books |
|||||||||||||||
1 |
J. Han and M. Kamber, "Data Mining: Concepts and Techniques", Morgan Kaufman |
||||||||||||||
Reference Books |
|||||||||||||||
1 |
Alex Berson, Stephen J. Smith, "Data Warehousing, Data Mining, and OLAP", MGH |
Course Outcomes: |
||||||||||||||||||||||||||||||||||||||||||||||||||||||||||||||||||
COs |
Description |
|||||||||||||||||||||||||||||||||||||||||||||||||||||||||||||||||
CO1 |
Recognize the process of data cleaning and pre-processing for mining applications |
|||||||||||||||||||||||||||||||||||||||||||||||||||||||||||||||||
CO2 |
Apply various frequent pattern mining techniques on various data and understand the importance of Attribute Selection |
|||||||||||||||||||||||||||||||||||||||||||||||||||||||||||||||||
CO3 |
Distinguish problems related to classification or clustering |
|||||||||||||||||||||||||||||||||||||||||||||||||||||||||||||||||
CO4 |
Evaluate accuracy of various algorithms and select appropriate method for their respective data. |
|||||||||||||||||||||||||||||||||||||||||||||||||||||||||||||||||
Mapping of CO and PO:
|