President Award Click here CAREER Click here
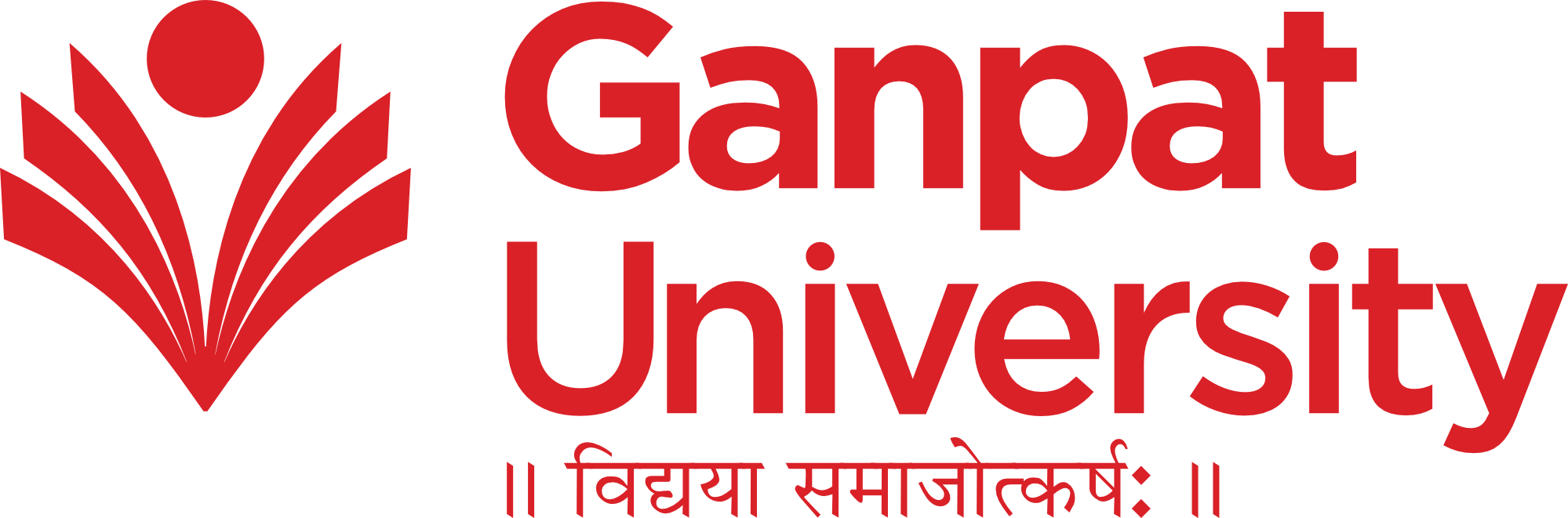
President Award Click here CAREER Click here
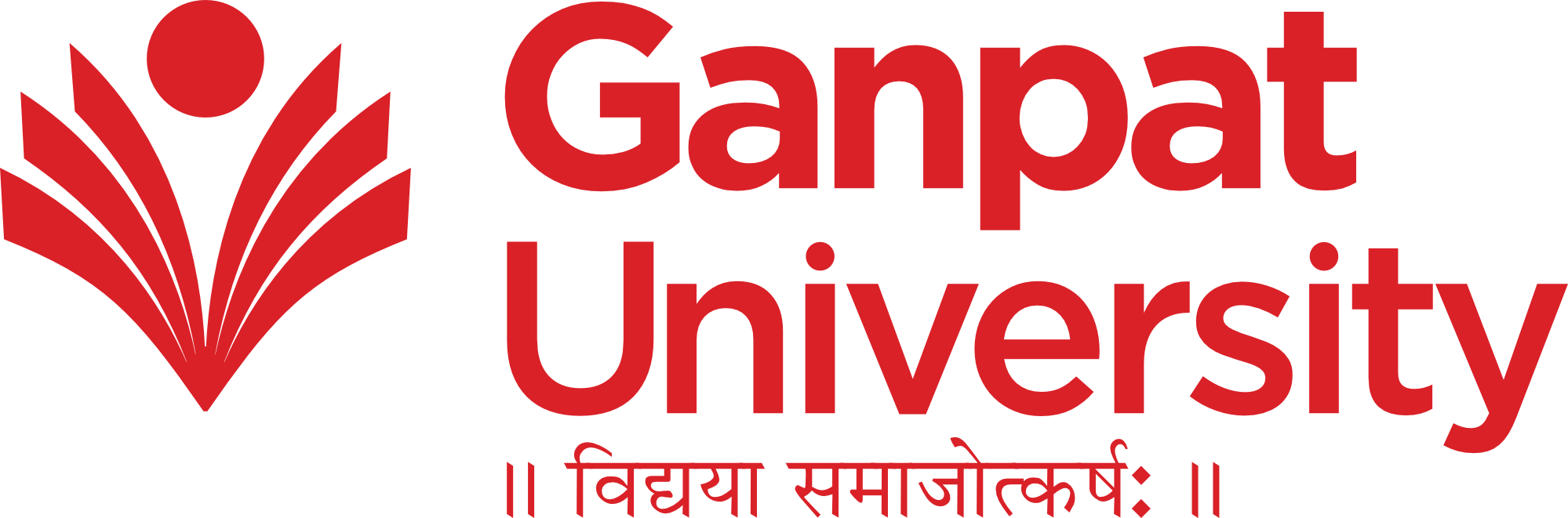
ADMISSION ENQUIRY - 2025
Machine Learning
GANPAT UNIVERSITY |
|||||||||||||||
FACULTY OF ENGINEERING AND TECHNOLOGY |
|||||||||||||||
Programme |
Bachelor of Technology |
Branch/Spec. |
Computer Science & Engineering (BDA/CBA) |
||||||||||||
Semester |
VII |
Version |
1.1.0.2 |
||||||||||||
Effective from Academic Year |
2022-23 |
Effective for the batch Admitted in |
June 2019 |
||||||||||||
Subject code |
2CSE702 |
Subject Name |
MACHINE LEARNING |
||||||||||||
Teaching scheme |
Examination scheme (Marks) |
||||||||||||||
(Per week) |
Lecture (DT) |
Practical (Lab.) |
Total |
CE |
SEE |
Total |
|||||||||
L |
TU |
P |
TW |
||||||||||||
Credit |
2 |
0 |
1 |
0 |
3 |
Theory |
40 |
60 |
100 |
||||||
Hours |
2 |
0 |
2 |
0 |
4 |
Practical |
30 |
20 |
50 |
||||||
Pre-requisites: |
|||||||||||||||
Probability theory and Bayesian Concept Learning, Statistics and data science, Algorithm analysis and design, Data Mining. |
|||||||||||||||
Learning Outcome: |
|||||||||||||||
After successful completion of the course, students will be able to
|
|||||||||||||||
Theory syllabus |
|||||||||||||||
Unit |
Content |
Hrs |
|||||||||||||
1 |
Introduction: Machine Learning Foundations: Design of a Learning system - Types of machine learning, Applications of machine learning. |
1 |
|||||||||||||
2 |
Supervised Learning: Regression algorithms, Classification algorithms Unsupervised Learning: Clustering algorithms |
9 |
|||||||||||||
3 |
Dimensionality Reduction Introduction, Feature Selection and Feature Extraction, Principal Component Analysis, Decision Tree algorithms, Ensemble methods |
5 |
|||||||||||||
4 |
Neural Network: Neural Networks - Introduction, Perceptron Learning, Backpropagation, Initialization, Training & Validation, Parameter Estimation - MLE, MAP, Bayesian Estimation. |
5 |
|||||||||||||
5 |
Convolutional Neural Networks (CNN): Introduction, Convolutional Neural Network (ConvNet/CNN), Evolution and Convolution Operation in CNN, Architecture of CNN, Convolution Layer, Activation Function (ReLU), Pooling Layer, Fully Connected Layer, Dropout |
5 |
|||||||||||||
6 |
Sequential Networks: Sequence modeling using RNNs, Backpropagation through time, Long Short-Term Memory (LSTM), Bidirectional LSTMs |
5 |
|||||||||||||
Self-Study: |
|||||||||||||||
LeNet-5, AlexNet, ZFNet, VGGNet, GoogleNet, ResNet. |
|||||||||||||||
Practical contents |
|||||||||||||||
Practicals shall be based on supervised, unsupervised learning and deep learning |
|||||||||||||||
Mooc Course |
|||||||||||||||
Course Name: Introduction to Machine Learning Link: https://www.edx.org/course/machine-learning-with-python-a-practical-introduct |
|||||||||||||||
Text Books |
|||||||||||||||
1 |
Christopher Bishop, “Pattern Recognition and Machine Learning” Springer. |
||||||||||||||
2 |
Kevin P. Murphy, “Machine Learning: A Probabilistic Perspective”, MIT Press. |
||||||||||||||
Reference Books |
|||||||||||||||
1 |
EthemAlpaydin, “Introduction to Machine Learning”, MIT Press. |
||||||||||||||
2 |
Tom Mitchell, "Machine Learning", McGraw-Hill. |
||||||||||||||
3 |
Trevor Hastie, Robert Tibshirani, Jerome Friedman, "The Elements of Statistical Learning", Springer. |
||||||||||||||
4 |
Stephen Marsland, “Machine Learning - An Algorithmic Perspective”, Chapman and Hall/CRC Press. |
||||||||||||||
Course Outcomes: |
||||||||||||||||||||||||||||||||||||||||||||||||||||||||||||||||||
COs |
Description |
|||||||||||||||||||||||||||||||||||||||||||||||||||||||||||||||||
CO1 |
Learn various machine learning approaches |
|||||||||||||||||||||||||||||||||||||||||||||||||||||||||||||||||
CO2 |
Learn different dimensionality reduction techniques |
|||||||||||||||||||||||||||||||||||||||||||||||||||||||||||||||||
Apply theoretical foundations of decision trees to identify best split and Bayesian classifier to label data points. |
||||||||||||||||||||||||||||||||||||||||||||||||||||||||||||||||||
CO4 |
Apply various classifier models like SVM, Neural Networks and identify classifier models for typical machine learning applications. |
|||||||||||||||||||||||||||||||||||||||||||||||||||||||||||||||||
Mapping of CO and PO:
|